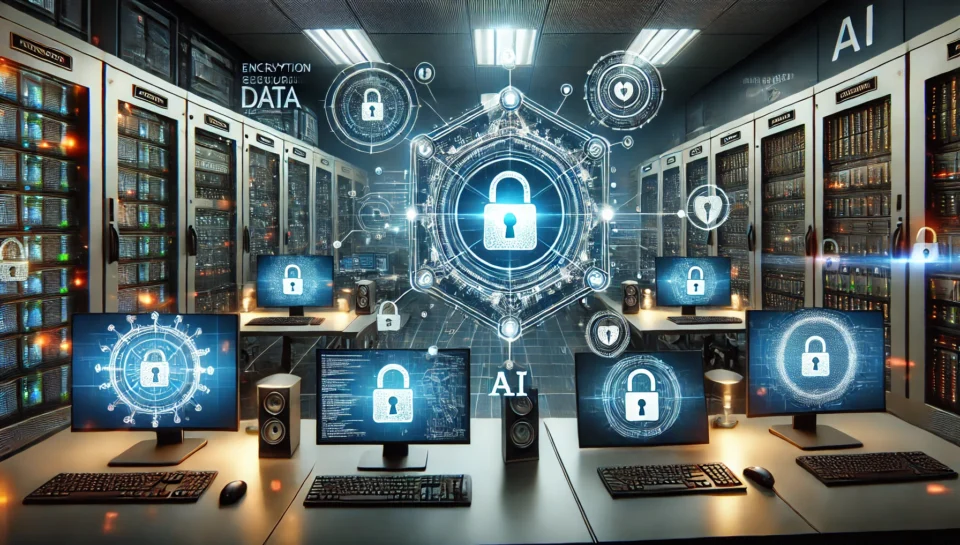
AI Automation and Data Security- What You Need to Know
As businesses increasingly adopt AI automation to enhance efficiency and productivity, ensuring data security becomes a critical concern. AI systems process vast amounts of data, making them potential targets for cyberattacks. This article explores
AI automation & data security: insights to protect your data.
Quick Navigation to Data Security in AI Automation
- Understanding AI Automation and Data Security: Key concepts and concerns.
- Common Security Risks: Identifying potential threats.
- Best Practices for Data Security: Protecting your data in AI systems.
- Implementing AI Security Solutions: Tools and technologies.
- Case Studies: Real-world examples of AI and data security.
- Future Trends: Emerging developments in AI data security.
- Ensuring Compliance: Meeting regulatory requirements.
Understanding AI Automation and Data Security: Key Concepts and Concerns
AI automation involves the use of artificial intelligence to automate routine tasks and processes. While AI offers significant benefits, it also raises data security concerns. AI systems rely on large datasets to function effectively, making data security a critical aspect of AI deployment.
Key Concerns:
- Data breaches and unauthorized access.
- Data integrity and accuracy.
- Privacy and compliance with regulations.
Benefits of Addressing Security:
- Protects sensitive information.
- Ensures data integrity and reliability.
- Builds trust with customers and stakeholders.
Common Security Risks: Identifying Potential Threats
Understanding the common security risks associated with AI automation helps in implementing effective safeguards.
- Data Breaches: Unauthorized access to sensitive data can lead to significant financial and reputational damage.
Example: Hackers exploiting vulnerabilities in an AI system to steal customer data.
- Insider Threats: Employees with access to AI systems might misuse data for personal gain or malicious purposes.
Example: A disgruntled employee tampering with AI algorithms to disrupt operations.
- AI Model Attacks: Adversaries manipulating AI models by feeding them malicious data to alter their behavior.
Example: Attackers providing skewed data to an AI system to influence its decision-making process.
- Data Privacy Violations: Improper handling of personal data can result in violations of privacy regulations such as GDPR or CCPA.
Example: An AI system inadvertently processing personal data without proper consent.
Best Practices for Data Security: Protecting Your Data in AI Systems
Implementing robust data security practices is essential to protect your AI systems and the data they process.
- Data Encryption: Encrypt sensitive data both at rest and in transit to prevent unauthorized access.
Example: Using SSL/TLS for secure data transmission and AES for data storage encryption.
- Access Controls: Implement strict access controls to ensure only authorized personnel can access sensitive data and AI systems.
Example: Using multi-factor authentication (MFA) and role-based access control (RBAC) to limit access.
- Regular Audits: Conduct regular security audits and vulnerability assessments to identify and address potential security gaps.
Example: Performing quarterly security audits to ensure compliance with security policies.
- Data Anonymization: Use data anonymization techniques to protect personal data while still enabling AI analysis.
Example: Anonymizing customer data before using it to train AI models.
- Secure Development Practices: Follow secure coding practices and conduct regular code reviews to minimize vulnerabilities in AI systems.
Example: Implementing secure coding guidelines and using static code analysis tools.
Implementing AI Security Solutions: Tools and Technologies
Several AI security solutions can help protect your data and AI systems.
- AI-Powered Security Tools: Leverage AI to enhance your security posture by detecting anomalies and responding to threats in real-time.
Example: Darktrace uses machine learning to identify and respond to cyber threats autonomously.
- Data Loss Prevention (DLP) Tools: Use DLP tools to monitor and control the movement of sensitive data within your organization.
Example: Symantec DLP helps prevent data breaches by monitoring and protecting sensitive information.
- Security Information and Event Management (SIEM) Systems: SIEM systems collect and analyze security data from various sources to detect and respond to threats.
Example: Splunk provides real-time insights and advanced threat detection capabilities.
Case Studies: Real-World Examples of AI and Data Security
- Financial Services: JPMorgan Chase uses AI to detect fraudulent activities and protect sensitive financial data. AI algorithms analyze transaction patterns to identify anomalies and prevent fraud.
- Healthcare: Mayo Clinic implements AI to enhance patient care while ensuring data security. AI-driven systems analyze medical data while adhering to strict privacy regulations.
- E-commerce: Amazon utilizes AI to optimize its supply chain and customer service operations. AI security tools monitor for potential threats and ensure the protection of customer data.
Future Trends: Emerging Developments in AI Data Security
- Explainable AI (XAI): Increasing transparency in AI decision-making processes to ensure security and trust.
Example: XAI techniques that provide clear explanations of AI decisions, helping identify and mitigate biases or vulnerabilities.
- Federated Learning: Enhancing privacy by training AI models across decentralized data sources without sharing raw data.
Example: AI models trained on-device across multiple users, reducing the need to centralize sensitive data.
- AI-Driven Security Analytics: Using advanced AI algorithms to predict and prevent security breaches.
Example: Predictive analytics tools that analyze vast amounts of data to identify potential security threats before they occur.
Ensuring Compliance: Meeting Regulatory Requirements
Compliance with data protection regulations is essential for businesses using AI.
- GDPR Compliance: Ensure your AI systems adhere to GDPR requirements, including data processing, storage, and consent.
Example: Implementing data protection impact assessments (DPIAs) for AI projects involving personal data.
- CCPA Compliance: Adhere to CCPA guidelines for data privacy, ensuring transparency and control over personal data usage.
Example: Providing customers with clear information on data collection and offering opt-out options.
- Industry-Specific Regulations: Comply with regulations specific to your industry, such as HIPAA for healthcare or PCI DSS for payment processing.
Example: Implementing encryption and access controls to protect healthcare data in compliance with HIPAA.
Insights from the Desk of EOcean Digital
AI automation offers immense benefits for businesses, but it also brings significant data security challenges. By understanding these challenges and implementing best practices, businesses can safeguard their data while leveraging AI’s full potential. At EOcean Digital, we are committed to helping businesses navigate the complexities of AI and data security. Embrace AI with confidence, knowing your data is protected and your operations are secure.
Protect your data while leveraging the power of AI with EOcean Digital’s expert solutions. Our team is here to help you implement robust data security measures and ensure compliance with regulations. Contact us today to get started!